Population health analytics is the systematic examination of health data of a population to identify and address factors that may be causing problems with population health and well-being. One of the earliest recorded instances of population health measurement was in 15th century England, where between 1665-1666, authorities in the city of London used death records to determine the impact of the excess deaths caused by the great plague on the city. The population health framework focuses more on ways to treat entire populations of people, rather than single individuals.
Modern healthcare relies on population health data analytics for effective care across different demographic elements. Employee clinical data collected from electronic health records, biometric screening, and health screening forms enhances the quality of care and directs specialized care to the correct individuals, ensuring better patient outcomes and lower healthcare costs.
Investing in population health analytics is a smart choice. Learn how implementing population health measures can improve your employee community health.
- What is Population Health Analytics?
- How is Population Health Data collected?
- What are the benefits of Population Health Analytics?
- What are the 4 main components of a Population Health Model?
- How is Population Health Analytics used to Improve Population Health?
- What are the main indicators of Population Health?
- Conclusion
What is Population Health Analytics?
Population health analytics enables efficient healthcare delivery by using data to identify population subgroups with similar health challenges or peculiar care needs and creating personalized care management for affected individuals. The data also focus on larger groups or communities with a shared medical problem to develop effective interventions within those populations.
Population health analytics improves physicians’ and healthcare providers’ ability to access data relating to medical history, including the care of individual patients and larger population groups. And it is a critical tool for tracking community health performances and understanding health behavior patterns across populations. Investing in healthcare analytics establishes the best disease prevention, chronic disease management, treatment, and self-care practices within a set population, i.e., your employees.
According to Dr. Kimberly Gobbins, a clinical faculty member of Southern New Hampshire University’s graduate nursing program. The ultimate goal of population health analytics is interpreting data on health risks and needs of populations and leveraging partnerships to data to improve their care and achieve positive health outcomes.
How is Population Health Data collected?
Data gathering from multiple sources is the backbone of any population health management system. However, integrating all the data into a single structure for easy quick and easy interpretation by physicians and providers is also vital. The main sources of population health data in the United States come from individuals (self-administered questionnaires), healthcare facilities and providers (medical records), the environment, and payers (claims).
The different methods for collecting population health data from the above sources include.
- Surveys – are the most common method for gathering population health information, and it is a structured and systematic method of collecting health data from a population sample. Researchers use the empirical data from surveys to understand, generalize or describe the entire population quantitatively.
- Medical records – are detailed and accurate data tracking patients’ health history, typically containing information on diagnosis, laboratory examinations, physician reports, etc.
Sources of medical records include
- Hospitals.
- Physician offices.
- Laboratories.
- Outpatient departments.
- Emergency departments.
- Inpatient settings.
- Claims (administrative) data – are administrative records (primarily electronic) containing information recorded directly by a healthcare provider. Claims data are from millions of doctor’s appointments, insurance information, medical bills, etc.
- Vital records – include records of births, deaths, marriages, and fetal deaths collected by the National Vital Statistics System and kept by state and local authorities. Vital records also provide valuable data on the cause of death, including rare disorders ending in death and birth details.
- Surveillance & disease registries – public health surveillance is the study of infectious diseases within a population. Primary sources of public health surveillance data are hospitals (private and public), laboratories, and care homes required by law to report incidences of specific diseases. Institutions (CDC, WHO) responsible for tracking and monitoring disease outbreaks analyze, store, and operate data gathered from surveillance activities and share them with local, state, and federal departments to organize control and intervention efforts.
Registries database systems maintained by public authorities, private groups, and nonprofits contain information about populations with certain diseases or conditions. They store data gathered from public health surveillance activities allowing researchers to track outbreaks of rare diseases over time and better understand their effects on population groups. Registries also offer information on improved care procedures and new effective treatment methods for specific conditions.
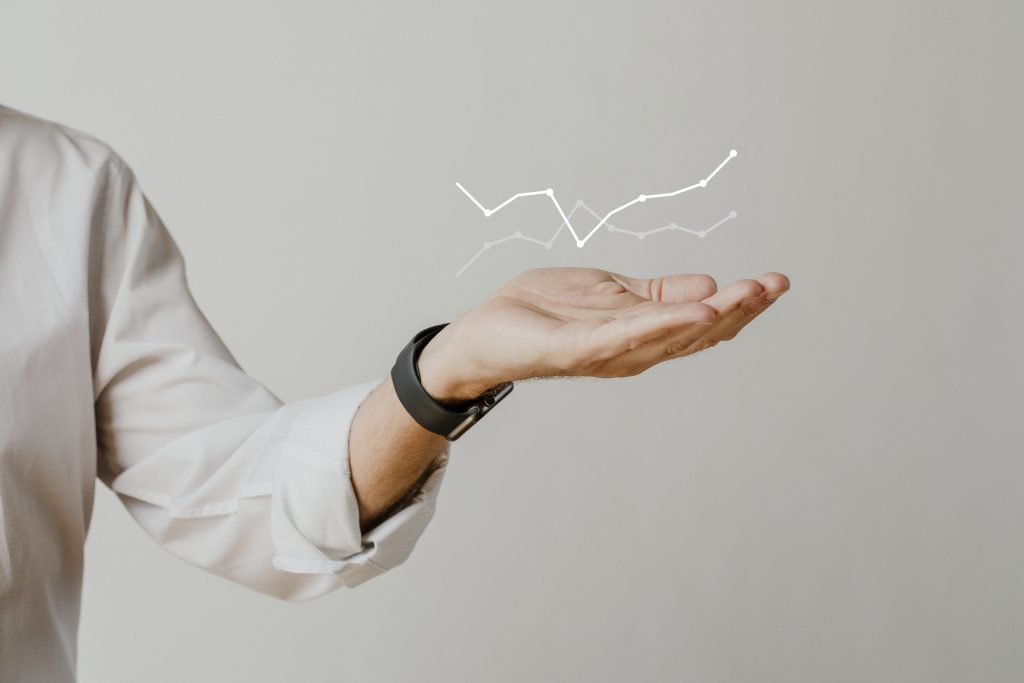
What are the 3 main purposes of Population Health Measurement?
In an era of increasing public health advocacy and awareness, the demand for better patient satisfaction and healthcare outcomes from healthcare organizations, including managed care plans, is expanding. Population health measurement aims to promote more public disclosure from medical groups and offer consumers increased access to comparative data when choosing providers.
The three main purposes of population health management include.
- Improvement – obtaining baseline data during the measurement process can help teams identify the problems and areas or problems requiring extra attention; or care opportunities that need improvement.
- Accountability – population health measurements reveal data on healthcare outcomes and cost of care, which allows healthcare purchasers and consumer advocates to demand greater assurances on the quality of care from healthcare providers.
- Research – although research is often time-consuming and expensive as a measure of population health measurement. It enables improved quality of care processes and reduces healthcare costs in hospitals and clinics.
What are the benefits of Population Health Analytics?
Population health analytics offers several benefits for payers and their provider networks, including access to vast amounts of standardized and accurate data allowing actionable insights which improve their decision-making plans. Below are some main advantages of population health analytics.
- Ability to execute quality improvement initiatives: Access to comprehensive employee medical data allows healthcare plans to develop accurate risk profiles and execute effective intervention plans resulting in better patient outcomes.
- Better resource allocation: Population health data promotes a better understanding of the disparities in patient care and helps payers, managed care plans, and healthcare providers to compare outcomes and allocate resources to areas where they can have the biggest impact. Some employers even conduct specific types workplace health promotions to address key pillars of wellness, based off of their population health data analysis.
- Ability to benchmark performance: Population health analytics provides information that payers often use to compare performances between different organizations. It also allows monitoring of the quality of care and influences a company’s decision in choosing a provider or managed care plan.
- Ability to predict patient risk: A huge benefit of population health analytics is that payers and healthcare providers can create detailed patient risk profiles from the data gathered. This information can help them deliver effective care interventions, particularly to patients with severe mental health issues (it’s also important for mental health in the workplace), life-threatening medical conditions, and substance abuse challenges.
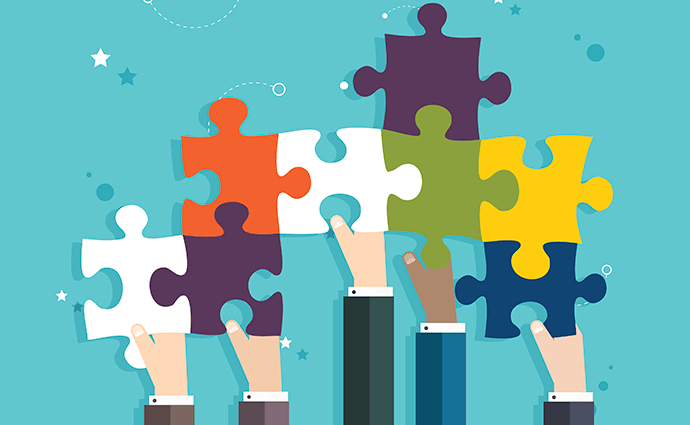
What are the 4 main components of a Population Health Model?
Developing a viable population health model requires certain critical elements. According to the National Committee for Quality Assurance, the four main elements of a population health model include.
- Focus on primary care.
Encouraging patients to take more responsibility for their lifestyle decisions and maintain healthy behaviors is a core element of population health models. This ensures patients take steps that ensure their overall well-being, like adopting healthy diets and engaging in fitness regimens instead of depending on treatment by physicians and caregivers for better health outcomes.
- Data-driven decision-making environment.
Population health models often use vast amounts of data gathered from healthcare providers. Electronic medical records from different sources can be integrated into a single database, making it easier for medical organizations to track their progress and identify areas of improvement in their care processes and patient health outcomes.
- Physician engagement.
Physicians are frontline caregivers within the healthcare industry. They determine patient treatments and level of care while accounting for more than a third of healthcare costs. Developing viable population health management initiatives gives physicians leadership roles allowing deep engagement with managed healthcare delivery systems.
- Pre and post-care disease management.
A major advantage of initiating population health models is focusing on patient care needs outside the hospital walls. It enables a system that tracks patients’ medication and diet requirements compliance.
How is Population Health Analytics used to Improve Population Health?
Using data to enhance patient outcomes is an objective of population health management. As healthcare providers search for cost-saving solutions while improving the quality of care, population health analytics can help can payers and managed care plans overcome the challenges of implementing not only thriving population health management systems but also identifying ways to improve them.
Population health analytics can improve population health in the following ways.
- Using insurance claims data to predict healthcare costs in a population health management system.
- Using electronic health records to improve the deficiencies in claims data collection.
- Using socioeconomic data to determine the impact of social problems on individual patients in a population health model.
- Using information from e-prescribing databases to track patients’ medication adherence, predict the possibility of a patient stopping their medication, and identify why they stopped.
What are the main indicators of Population Health?
Population health indicators offer insight into the quality of population health. They measure specific health attributes within a population, provide data to track disease prevalence, and develop solutions to improve population health.
The main indicators of population health include.
- Morbidity Indicators.
Morbidity indicators measure incidences or prevalence of diseases, disabilities, or injuries within populations. Sources of data on mobility rates include hospital/outpatient clinic records on disease outbreaks, direct surveys, population surveillance, and registries.
- Mortality Indicators.
These are indicators measuring death rates in a population. Mortality indicators measure the severity and intensity of a disease ravaging a population by the number of deaths recorded.
Examples of mortality indicators used to measure population death rates include.
- Crude Death Rate (CDR).
- Infant mortality rate.
- Maternal mortality ratio.
- Mortality from acute respiratory infection in children under age five.
- Proportional mortality from ill-defined causes.
- Indicators of behavioral risk factors.
Changes in lifestyle habits in the past few decades have resulted in the increasing occurrence of chronic non-communicable diseases (CNCD) like lung cancer and diabetes, which currently occur more than infectious diseases in a population. To enable health authorities to perform effective health surveillance practices, health authorities have identified modifiable behavioral risk factors associated with CNCDs to promote lifestyle changes that can reduce incidences of CNCDs in a population.
Examples of such modifiable behavioral risk factors include.
- Sedentary lifestyle.
- High tobacco consumption rates.
- Adopting unhealthy diets.
- High alcohol consumption rates.
- Health Services Indicators.
These are conceptual frameworks designed to create new and more advanced indicators measuring the quality of healthcare services in a population while acknowledging the increasing challenges in healthcare delivery.
Conclusion
Investing in a comprehensive public health management system requires a solid database foundation providing accurate analysis. Kindly call one of our experts if you are interested in working with IncentFit. We will work together to develop your company’s best public health management technology.